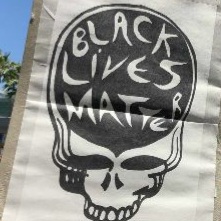
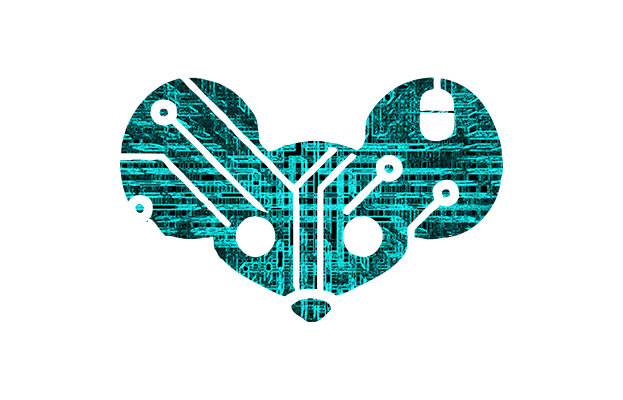
This regulation (and similar being proposed in California) would not be applied retroactively.
This regulation (and similar being proposed in California) would not be applied retroactively.
https://arewereorganizedyet.com/ lol already updated
How does lemmy federation work in this case? Conceivably after being restored from backup the lemmy.ml instance could see those few hours of lost history as federated to other lemmy instances and resync it back as the host instance. Obv I’m vastly oversimplifiying things but what happens today?
https://github.com/Mozilla-Ocho/Memory-Cache is the actual project if you want to use it.
Basically it’s a firefox extension to save a page as a pdf in a directory that is symlinked to your local PrivateGPT install which then ingests the docs. It doesn’t seem to me that it provides any in-browser querying of PrivateGPT but I haven’t tried setting it up to confirm that.
Re thumb-key do you have recommended tutorials for getting comfortable with it? I found trying to do touch typing tutorials didn’t really help, both because they are generally made for desktop environments and they are geared towards qwerty layout (e.g., get comfortable with home row first etc). I tried forcing myself to use it for a full 24 hours as the concept makes a ton of sense to me, but got very frustrated with myself and then dug into the world of which layout to choose, got overwhelmed, and switched back to whatever this qwerty layout that samsung one ui provides on galaxys.
I think that is overly simplistic. Embeddings used for LLMs do definitely include a concept of what things mean and the relationship of things to other things.
E.g., compare the embeddings of Paris, Athens, and London to other cities and they will have small cosine distance between them. Compare France, Greece, and England and same. Then very interestingly, look at Paris - France, Athens - Greece, London - England and you’ll find the resulting vectors all align (fundamentally the vector operation seems to account for the relationship “is the capital of”). Then go a step further, compare those vector to Paris - US, Athens - US, London - Canada. You’ll see the previous set are not aligned with these nearly as much but these are aligned with each other (relationship being something like “is a smaller city in this countrry, named after a famous city in some other country”)
The way attention works there is a whole bunch of semantic meaning baked into embeddings, and by comparing embeddings you can get to pragmatic meaning as well.
I agree. Family of 5 many hotels require us get 2 rooms. Plus no option to cook meals makes for a much more expensive stay usually. At least until a few years ago when airbnb went insane with the cleaning fees plus cleaning requirements and all that nonsense.
Many (14?) years back I attended a conference (now I can’t remember what it was for, I think a complex systems department at some DC area university) and saw a lady give a talk about using agent based modeling to do computational sociology planning around federal (mostly navy/army) development in Hawaii. Essentially a sim city type of thing but purpose built to help aid in public planning decisions. Now imagine that but the agents aren’t just sets of weighted heuristics but instead weighted heuristic/prompt driven LLMs with higher level executive prompts to bring them together.
I fully agree with this, would have written something similar but was eating lunch when I made my former comment. I also think there’s a big part of pragmatics that comes from embodiment that will become more and more important (and wish Merleau-Ponty was still around to hear what he thinks about this)
A lot of semantic NLP tried this and it kind of worked but meanwhile statistical correlation won out. It turns out while humans consider semantic understanding to be really important it actually isn’t required for an overwhelming majority of industry use cases. As a Kantian at heart (and an ML engineer by trade) it sucks to recognize this, but it seems like semantic conceptualization as an epiphenomenon emerging from statistical concurrence really might be the way that (at least artificial) intelligence works
The title suggests per day to me but the graph I’m quite sure is cumulative based on other data
I live upon morsels you happen to drop